Hi👋, I am currently pursuing a PhD at the State Key Laboratory of Multimodal Artificial Intelligence System, Institute of Automation, Chinese Academy of Sciences (CASIA), under the guidance of Prof. Weiming Hu, Prof. Bing Li, Prof. Chunfeng Yuan and Associate Prof. Juan Wang.
Before that, I got my bachelor’s degree from Beijing Jiaotong University (BJTU) in 2022. Especially thank Prof. Runming Cong and Prof. Fangshi Wang for their guidance during this period.
My research interests include:
- Computational Imaging
- Image Quality Assessment (IQA)
- Image Signal Processing (ISP)
- Large Language Model (LLM)
I am trying to explore and solve some intersting challenges in these fields. I have published some papers at the top international AI conferences (ECCV, IJCV, ACCV, etc.) .
📫 My email: chencn2018@163.com, chenzewen2022@ia.ac.cn
🏁 My Research Repos
- Image Quality Assessment
🔥 News
-
🎉 07/2024: our work “PromptIQA: Boosting the Performance and Generalization for No-Reference Image Quality Assessment via Prompts” was accepted by The 18th European Conference on Computer Vision (ECCV 2024).
-
🎉 08/2023: we won the second prize in the “AIGC Inference Performance Optimisation Track” held by Baidu.
-
🎉 07/2023: our work “Hierarchical Curriculum Learning for No-reference Image Quality Assessment” was accepted by International Journal of Computer Vision (IJCV).
-
🎉 09/2022: our work “Teacher-Guided Learning for Blind Image Quality Assessment” was accepted by The 16th Asian Conference on Computer Vision (ACCV 2022).
📝 Publications
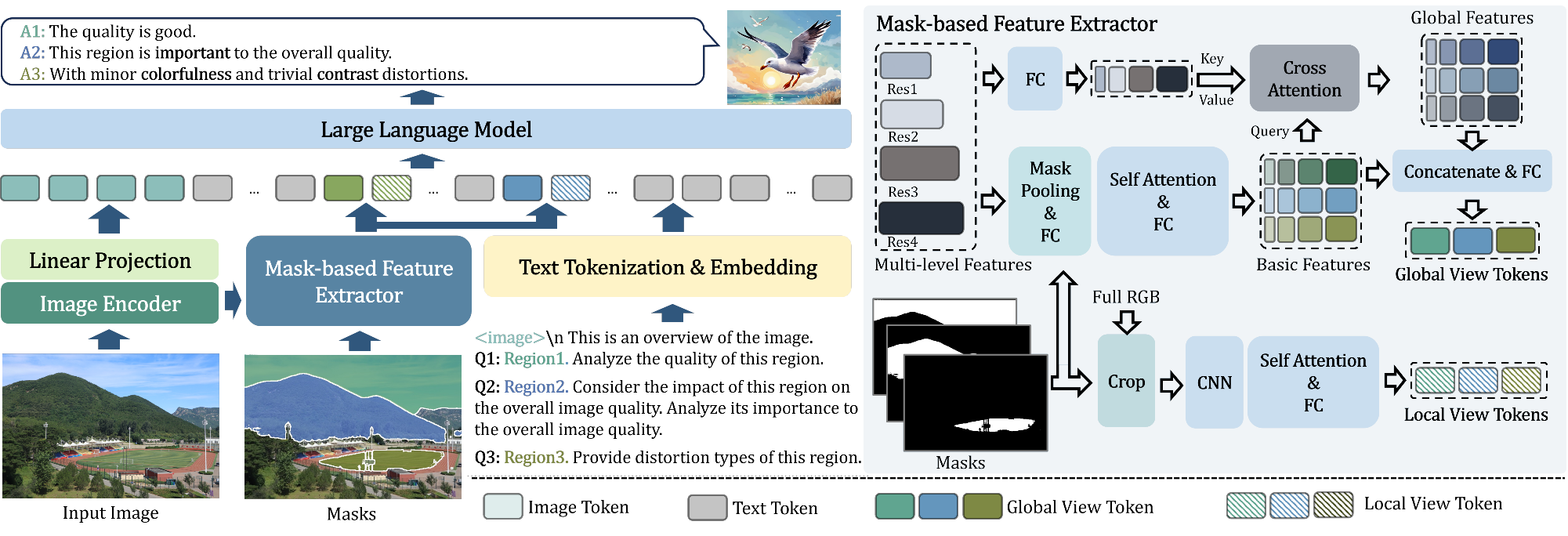
Zewen Chen, Juan Wang, Wen Wang, Sunhan Xu, et al.
TL;DR: We propose a novel network (SEAGULL) and construct two datasets (SEAGULL-100w and SEAGULL-3k) to achieve fine-grained IQA for any ROIs.
Quick Read (Click Me)
Existing Image Quality Assessment (IQA) methods achieve remarkable success in analyzing quality for overall image, but few works explore quality analysis for Regions of Interest (ROIs). The quality analysis of ROIs can provide fine-grained guidance for image quality improvement and is crucial for scenarios focusing on region-level quality. This paper proposes a novel network, SEAGULL, which can SEe and Assess ROIs quality with GUidance from a Large vision-Language model. SEAGULL incorporates a vision-language model (VLM), masks generated by Segment Anything Model (SAM) to specify ROIs, and a meticulously designed Mask-based Feature Extractor (MFE) to extract global and local tokens for specified ROIs, enabling accurate fine-grained IQA for ROIs. Moreover, this paper constructs two ROI-based IQA datasets, SEAGULL-100w and SEAGULL-3k, for training and evaluating ROI-based IQA. SEAGULL-100w comprises about 100w synthetic distortion images with 33 million ROIs for pre-training to improve the model's ability of regional quality perception, and SEAGULL-3k contains about 3k authentic distortion ROIs to enhance the model's ability to perceive real world distortions. After pre-training on SEAGULL-100w and fine-tuning on SEAGULL-3k, SEAGULL shows remarkable performance on fine-grained ROI quality assessment.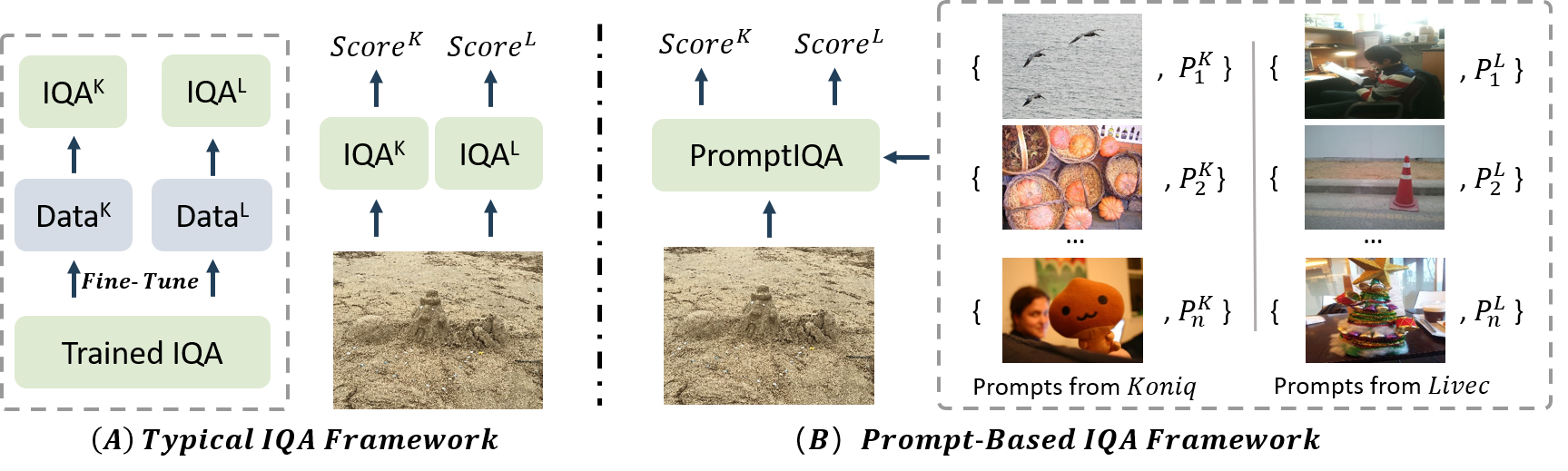
Quick Read (Click Me)
Due to the diversity of assessment requirements in various application scenarios for the IQA task, existing IQA methods struggle to directly adapt to these varied requirements after training. Thus, when facing new requirements, a typical approach is fine-tuning these models on datasets specifically created for those requirements. However, it is time-consuming to establish IQA datasets. In this work, we propose a Prompt-based IQA (PromptIQA) that can directly adapt to new requirements without fine-tuning after training. On one hand, it utilizes a short sequence of Image-Score Pairs (ISP) as prompts for targeted predictions, which significantly reduces the dependency on the data requirements. On the other hand, PromptIQA is trained on a mixed dataset with two proposed data augmentation strategies to learn diverse requirements, thus enabling it to effectively adapt to new requirements. Experiments indicate that the PromptIQA outperforms SOTA methods with higher performance and better generalization.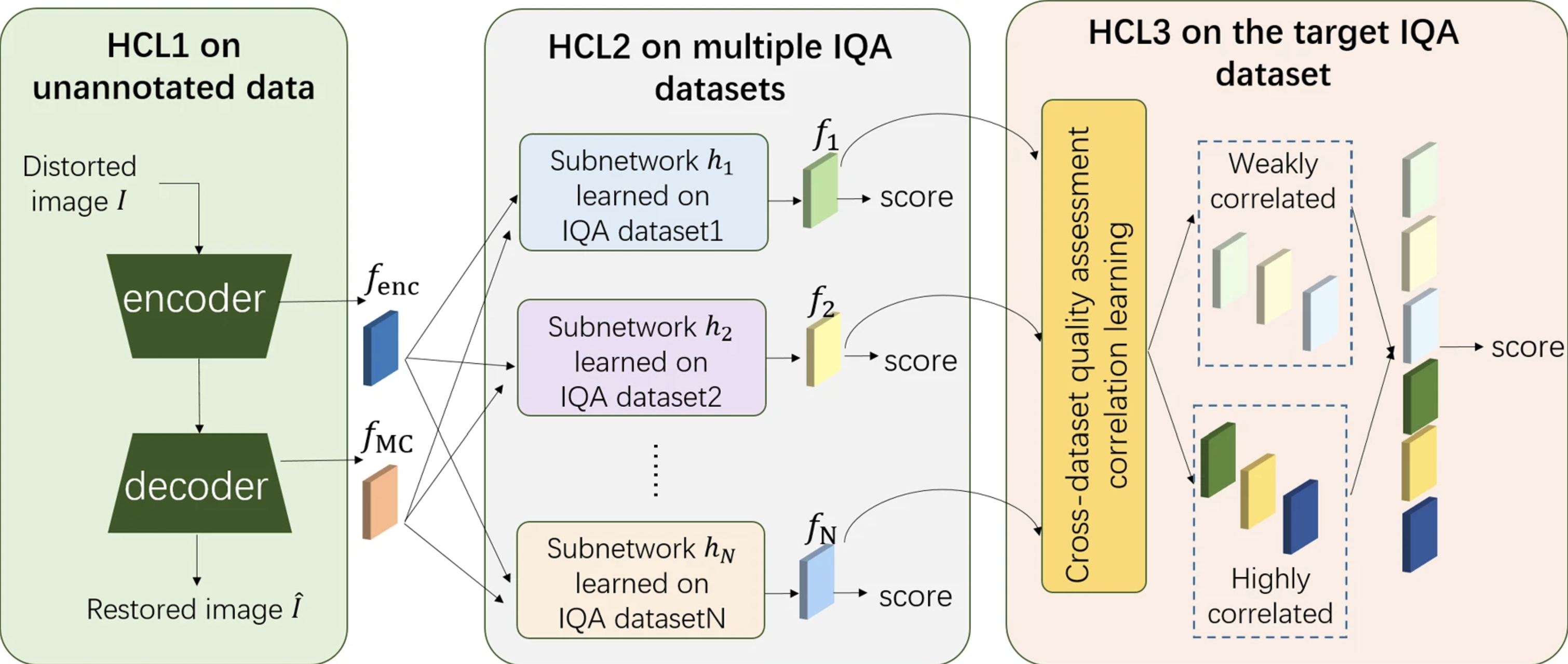
Juan Wang, Zewen Chen, Chunfeng Yuan et al. (Co-first author)
TL;DR: We develope a hierarchical curriculum learning (HCL) framework for NR-IQA by decomposing complex tasks into three levels: basic, intermediate, and professional to improve performance.
Quick Read (Click Me)
This work addresses the problem of insufficient labeled data for no-reference image quality assessment (NR-IQA) with the help of pre-training techniques and external unsupervised data. We design a hierarchical curriculum learning (HCL) framework for NR-IQA, which leverages the external data to learn the prior knowledge about IQA widely and progressively. Specifically, as a closely-related task with NR-IQA, image restoration is used as the first curriculum to learn the image quality related knowledge (i.e., semantic and distortion information) on massive distorted-reference image pairs. Then multiple lightweight subnetworks are designed to learn human scoring rules on multiple available synthetic IQA datasets independently, and a cross-dataset quality assessment correlation (CQAC) module is proposed to fully explore the similarities and diversities of different scoring rules. Finally, the whole model is fine-tuned on the target authentic IQA dataset to fuse the learned knowledge and adapt to the target data distribution. The experimental results show that the designed pre-trained model can achieve good prediction accuracy and generalisation.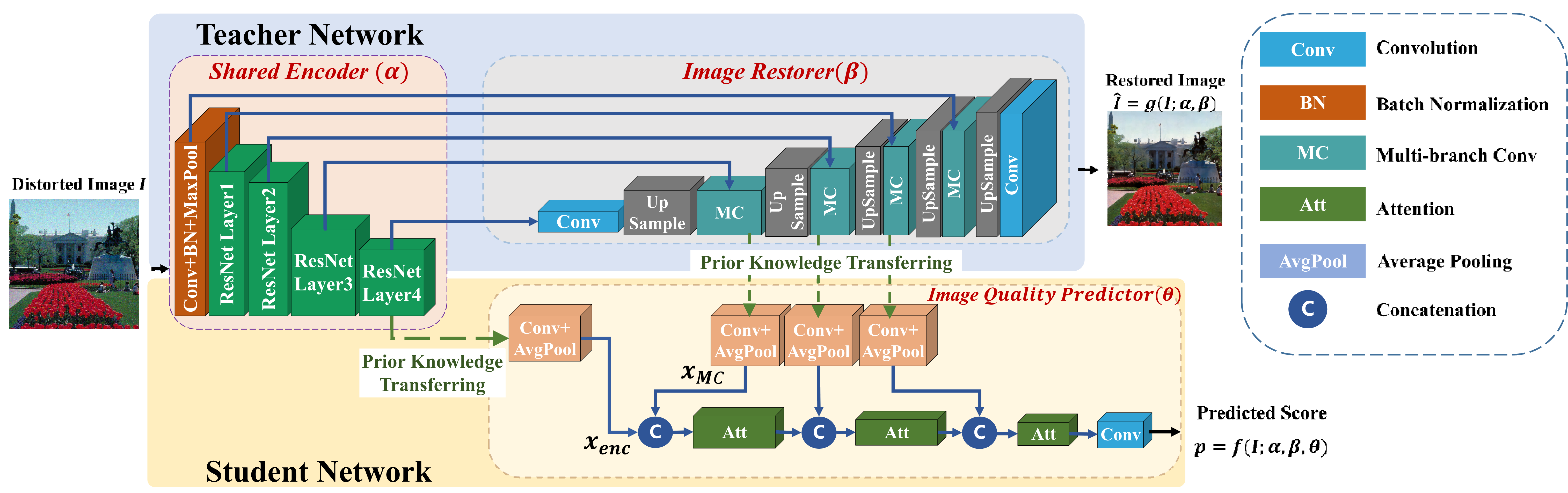
Zewen Chen, Juan Wang, Bing Li et al.
TL;DR: We introduce a novel NR-IQA framework that leverages an image restoration teacher network (TN) to transfer semantic and distortion knowledge to a student network (SN), enabling superior BIQA performance on limited annotated data with validated generalization, robustness, and effectiveness.
Quick Read (Click Me)
The performance of deep learning models for blind image quality assessment (BIQA) suffers from annotated data insufficiency. However, image restoration, as a closely-related task with BIQA, can easily acquire training data without annotation. Moreover, both image semantic and distortion information are vital knowledge for the two tasks to predict and improve image quality. Inspired by these, this paper proposes a novel BIQA framework, which builds an image restoration model as a teacher network (TN) to learn the two aspects of knowledge and then guides the student network (SN) for BIQA. In TN, multi-branch convolutions are leveraged for performing adaptive restoration from diversely distorted images to strengthen the knowledge learning. Then the knowledge is transferred to the SN and progressively aggregated by computing long-distance responses to improve BIQA on small annotated data. Experimental results show that our method outperforms many state-of-the-arts on both synthetic and authentic datasets. Besides, the generalization, robustness and effectiveness of our method are fully validated.🏆 Project Achievements
- 12/2024-Now : Image Representation Factor Prediction and Artifact Analysis
- TBU
- 06/2021-10/2024 : AI-Based Black Box ISP Hyperparameter Optimization
- TBU
- TBU
🎖 Honors and Awards
- 08/2023: The second prize in the “AIGC Inference Performance Optimisation Track” held by Baidu.
📖 Educations
- 2022.09 - Now PhD: State Key Laboratory of Multimodal Artificial Intelligence System, Institute of Automation, Chinese Academy of Sciences (CASIA)
- 2018.09 - 2022.06 Bachelor: School of Software, Beijing Jiaotong University (BJTU)